For a number of years now, a growing trend in online information retrieval has been “social Web search” (or simply “social search”). It’s a term that continues to be tweaked and defined, and there are some quite different interpretations of what social search actually means. Generally speaking folks interpret social search to mean a form of Web search informed by the searcher’s social graph. The way in which the social graph informs search tends to be searching social feeds from the likes of Facebook, Google Plus and – once upon a time – Twitter. Posts or status updates containing links that match the user’s query can be integrated with regular search results. In this post we explore some of the ramifications of this approach to social search and suggest a different way of looking at things.
The problem with searching the social Web
Sometimes I’m looking for information that a friend knows. Sometimes they’ve even posted a link to Facebook (if I’m searching on Bing) or Google+, liked it or +1’d it, all of which require explicit action or effort. The phenomenon of lurkers applies here, where by definition participation levels will be low on any service that relies on user-generated content. Though I almost always use it before I travel, if my TripAdvisor profile was used to decide what places I can be asked for advice about, it would show that i’m an authority on St. Patrick’s Cathedral in Dublin and nowhere else (see Figure 1). I’ve been to every continent except the Antarctic but I haven’t taken the time to add that information to TripAdvisor. That’s ok, i’m basically a lurker and I don’t have the motivations of the power users or even arguably the casual contributors – on TripAdvisor at least.
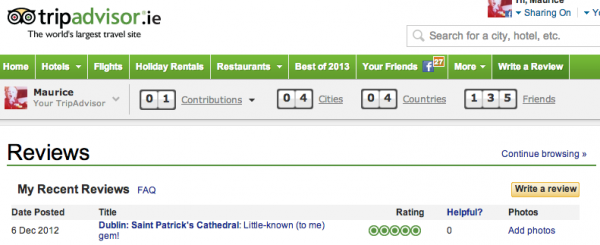
Figure 1: My TripAdvisor profile – containing a single lonely review – does not capture my full travelling experience.
The point is that even if one of my friends knows about the topic i’m searching on, the chances of them having posted it online are pretty slim. Any social search service that relies on this explicit interaction will suffer from a coverage problem. Though it will be very useful in some circumstances, its application will be limited.
Graphs, graphs everywhere
It seems like everything’s a graph these days. Social graphs, interest graphs, mood graphs, brand graphs, opinion graphs, even automotive graphs are being used to make sense of worlds both online and offline. Each kind of graph is a representation of the relationships between objects. For example, a social graph portrays connections between people and represents “who you know”, embodied most ubiquitously on Facebook. An interest graph such as those maintained by Twitter, Quora, Pinterest, represents “what you like”.
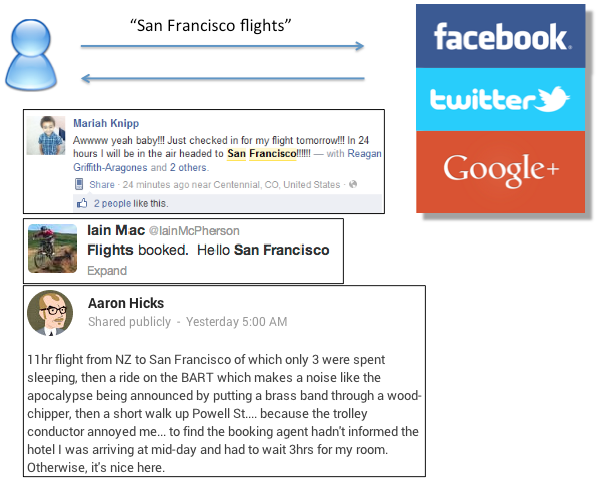
Figure 2: For the phrase “san francisco flights”, posts to social sites such as Facebook (1st), Twitter (2nd) or Google Plus (3rd) may not make suitable sources of search results.
The thing about using a searcher’s social graph to inform result rankings is that usually when I search I don’t care if it’s a friend who knows the answer – I just want the results. In fact, for most of my searching activity none of my Facebook friends knows about the topic i’m interested in. Even if they did there’s a limited chance they’ll be an authority on it. So which graph to use to drive social search, then? Interest graphs sound like promising candidates, since I want to see results that relate to my interests, ideally contributed by people who share those interests. We quickly encounter the problem outlined above that the interests that people choose to share on social sites may not reflect the full gamut of my search interests; certainly not in the depth I frequently need to search on for some search tasks. Most importantly, when people post to Facebook, Twitter and Google Plus they are unlikely to have the same underlying intent as we find in a search context (see Figure 2).
Social Search is a Process
At HeyStaks we place a large emphasis on search as a source of recommendations, something that is absent in other offerings. Our platform can accept explicit contributions such as tagging (and even link-containing status updates). However, the primary way in which HeyStaks decides which results are important for a given search need is by learning from the search activities (submitting queries and selecting results) of users.
Because search is a selfish activity, users are not motivated by public recognition, relationship building, attention-seeking or even altruism. Also, we search across many more topics that we are interested in on a daily basis than we will ever post about on social networks. The bottom line is that the queries we submit daily are rich with genuine intent. This is precisely why Google has been so successful with search advertising; it recognises that our queries are “honest signals” of future intent to act and so it can link us with service providers and sellers we want to interact with.
In addition, we focus more on the topics or interests that users express preference for than we do on actual connections between users. We link people and drive recommendations between them based on “what they know” not “who they know”, a kind of search interest graph to use the trendy nomenclature. Importantly, our patent-pending reputation model understands for each topic of interest, how good a person is at recommending content for others on that topic. This means that identifying experts is easier and more powerful than techniques that rely on self- or peer-nomination (remember, search is selfish!).
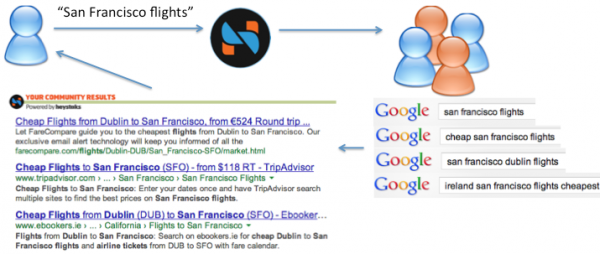
Figure 3: HeyStaks learns which users I share interests with, based on our searching histories. When I search for “san francisco flights”, the results selected for similar queries that these users have submitted can be recommended to me. Further, these results are ranked according to the reputation of experts (coloured orange here). I benefit from the previous efforts and more complex queries of like-minded, expert users so my own search experience is much better.
The result is a platform that delivers highly relevant, personalized results that are drawn from communities of users who share interests with the searcher, weighted by the relative expertise of those community members. All based fundamentally on actual search activity so our technique is not just a feature like some offerings are. Rather, it’s a process that transforms any search environment from an individual, self-driven task into a collaborative, peer- and expert-driven experience. The power of search relevance is placed firmly in the hands of users rather than solely with search engines and publishers.
The two main components of social search as we view it are that it must involve altering the actual process of search and it must involve people interacting together to solve the problem of search. In that regard we would be classified more as collaborative search, insofar as we deliver an entirely different mechanism for finding content online than simply searching the social Web.
Finally, a definition of social search that makes sense. Do you agree? What are your experiences with the social offerings that are currently available? We’d love to hear your thoughts in the comments!